PharmacoInformatics
Data-driven pharmacotherapy. We are dedicated to optimizing pharmacotherapy outcomes by leveraging information technology. Our primary focus is on patients with multiple chronic conditions and therefore taking multiple medications. Currently, there is limited knowledge available concerning the safety and effectiveness of pharmacotherapy in these patients. Consequently, these patients have a higher risk of medication-related harm and treatment failure.
To address this critical issue, we conduct analyses of real-world healthcare data. Our objective is to obtain real-world evidence regarding the safety and effectiveness of pharmacotherapy in patients with multiple chronic conditions. Subsequently, we translate these insights into digital tools. Tools designed to aid healthcare providers in prescribing and monitoring medications safely and effectively.
Scientific projects
The current best practice approach for the diagnosis of adverse drug events (ADEs) in hospitalized patients relies on a manual procedure, including a full patient chart review and a formal causality assessment by multiple medical experts. The resource intensive character of this approach, hampering large scale medication safety monitoring and use in daily, often busy, clinical practice. Furthermore, this approach also suffers from low inter-rater reliability and a high risk of bias. Novel methods for the diagnosis of ADEs are therefore urgently needed, and artificial intelligence may aid through automated, data-driven approaches. Within the hospitalized patients, patients admitted to the Intensive Care Unit, are most prone to ADEs due use of multiple, high-risk drugs. Especially drug-induced acute kidney injury (DAKI) presents serious concerns for ICU patients. Therefore, the aim of the RESCUE project is to develop and validate an automated DAKI causal algorithms for ICU patients by utilizing advanced machine learning techniques and best-practices for causal inference using observational routinely collected ICU data. If proven successful, the developed automated and data-driven approach may be applicable for any other ADEs.
Timespan: 2020-2024
Research line: PharmacoInformatics
Website: RESCUE
Adverse drug events (ADEs) are a major public health concern. In the Netherlands, ADEs are a leading cause of preventable patient harm in hospitalized patients, and their reduction is needed but so far unsatisfactory. Currently, information about ADE occurrence mainly comes from voluntary reporting. However, research shows that fewer than 1% of ADEs are reported in this way. ADEs are often documented by physicians in clinical notes as part of routine care, and clinical pharmacists would need to manually analyze vast amounts of clinical notes to gain a thorough insights on ADE occurrence. This is prohibitively time consuming and not future-proof. An automated method for ADE detection from clinical notes would support clinical pharmacists in their crucial role as medication safety gatekeepers. Natural language processing (NLP), a computerized approach to analyze text data, has shown promising results for the purpose of ADE detection from clinical notes. However, most NLP methods available were develop on clinical text in English.
Within the hospitalized patients, patients admitted to the Intensive Care Unit, are most prone to ADEs due use of multiple, high-risk drugs. At present, a routine identification of Adverse Drug Events (ADEs) is not available for Dutch Intensive Care Units (ICU). This hampers timely ADE detection. Especially drug-induced acute kidney injury (DAKI) presents serious concerns for ICU patients. Therefore, the main goal of the REMEDY study is to develop and validate an automated method to detect ADEs in Dutch clinical notes using Natural Language Processing (NLP).
Timespan: 2020-2024
Research line: PharmacoInformatics
Website: REMEDY
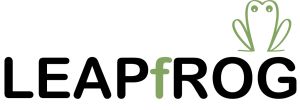
People with multimorbidity suffer from suboptimal pharmacotherapy due to insufficient knowledge to guide prescribing decisions. Leveraging routinely collected data in Electronic Health Records (EHRs) using machine learning (ML), have been widely advocated to close this knowledge gap and provide missing insights on safety and effectiveness of drugs. However, EHR data suffer from many quality issues. In addition, there are still significant limitations in the introduction of ML models to support in prescribing decisions, due to the lack of transparency in how model outputs are generated (the "black box” dilemma), and lack of logical reasoning (models not informed by causal domain knowledge).
Therefore, LEAPfROG aims for scientific breakthrough in ML application in healthcare by addressing the aforementioned limitation and creating a medication safety learning system. A system powered by novel ML-powered tools capable to provide trustworthy and explainable causal suggestions. The value of the ssystem will be demonstrated via clinically relevant and urgent use case of drug-induced kidney diseases (DIKD) in patients with chronic kidney disease (CKD). Patients with CKD are amongst the most complex and multimorbid. By providing missing insights on DIKD in patients with CKD via PIL, LEAPfROG will contribute to safer and more effective pharmacotherapy in these patients. Aiming to increase quality of life of patients with CKD and decrease healthcare costs related to medication harm
Timespan: 2022 - 2027
Research line: PharmacoInformatics
Website: LEAPfROG
Staff involved