On this page, you will find the ongoing scientific projects!
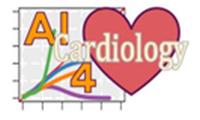
AI4Cardiology is a collaborative data science effort performed by a multidisciplinary team to explore the potential of machine learning in the field of cardiology. By delving deep into complex, real-world cardiac datasets, we aim to uncover valuable insights and positively impact clinical decision-making.
Time span: 2021-2024
Research line: Methods in Medical Informatics
Staff: Tseko Yordanov, Anita Ravelli, Ameen Abu-Hanna
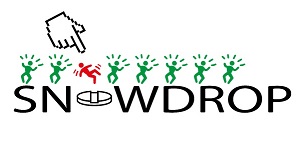
Within the SNOWDROP project, we are developing a clinical decision support system for decreasing fall risk-increasing medication for general practitioners. The system predicts the individual risk of falls for the older patient based on prediction models. If a patient has a high risk of falling according to the system, the GP can call the patient for a consultation in which a medication assessment will be carried out and the fall risk will be further identified. The patient is offered information about the fall risk and medication-related treatment options via a patient portal. The patient can use this information to prepare for the consultation. During the consultation, the clinical decision support system provides support to the GP and the patient for joint decision-making about adjusting fall risk-increasing medication. The patient can view the decisions and advice discussed during the consultation in the patient portal.
Timespan: 2019-2024
Research line: Methods in Medical Informatics
Staff: Ameen Abu-Hanna, Stephanie ''Ace'' Medlock, Noman Dormosh
Website: SNOWDROP
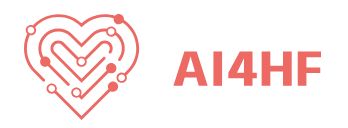
The AI4Hf project focusses on co-design, development, evaluation and exploitation of integrative and thrustworthy artificial intelligence solutions for personalized heart failure risk-assessment. These tools will be trained using large-scale complementary multi-source cardiovascular data including cardiac imaging, -biomarkers, electrocardiography and free text data. Within the project, great emphasis is placed on the concept of trustworthy and inter-dicilplinary tool design, to ensure that developed tools are trusted, approved and deployed in a real world setting and providing clear benefit for patients, cardiologists, physicians and health-care providers.
The consortium is led by Amsterdam UMC and Amsterdam UMC focusses on defining requirements for the development of trustworthy AI, model development and explainability.
Research line: Methods in Medical Informatics
Staff: Giovanni Cinà, Machteld Boonstra
Website: www.ai4hf.com
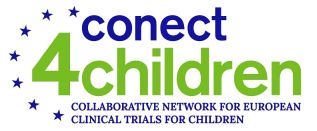
c4c (conect4children) is a large collaborative European network that aims to facilitate the development of new drugs and other therapies for the entire paediatric population. It is a pioneering opportunity to build capacity for the implementation of multinational paediatric clinical trials whilst ensuring the needs of babies, children, young people and their families are met.
c4c is committed to meeting the needs of paediatric patients thanks to a novel collaboration between the academic and the private sectors, which includes 35 academic and 10 industry partners and around 500 affiliated partners. c4c endeavours to provide a sustainable, integrated platform for the efficient and swift delivery of high quality clinical trials in children and young people across all conditions and phases of the drug development process. The project strives to bring innovative processes to all stages of clinical development by generating a new model of organization and of the clinical development process.
Research line: Reusable health data
Staff: Nirupama Benis, Ronald Cornet
Website: conect4children

The overall aim of CAPABLE is to combine the most advanced technologies for data and knowledge management with a sound socio-psychological approach in order to develop a coaching system for improving the quality of life of cancer home patients. The system aims at early detecting and managing cancer-related issues and at satisfying the needs of patients and their home caregivers.
Research line: Reusable health data
Staff: Ronald Cornet, Savannah Glaser, Rowdy de Groot, Stephanie ''Ace'' Medlock
Website:Capable
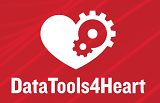
Within the datatools4heart project, we work on the co-creation, development and demonstration of a comprehensive, federated, privacy-preserved cardiology data toolbox. This toolbox includes standardized data ingestion and harmonization tools, multilingual natural language processing and federated machine learning and data synthesis methods. Additionally, with the development of a public data catalog combined with virtual assistants, scientist and clinicians can navigate easily through large-scale multi-source cardiology electronic health record data, while complying to the European regulations and data standards.
Amsterdam UMC participates as one of the clinical sites, focusing on data harmonization, adoption of a federated learning platform and free-text analysis using natural language tools.
Research line: Methods in Medical Informatics
Staff: Noman Dormosh, Iacer Coimbra Alves Cavalcanti Calixto, Ameen Abu-Hanna, Machteld Boonstra
Website: www.datatools4heart.eu

The European Joint Programme on Rare Diseases (EJP RD) is a programme aiming to create an effective rare diseases research ecosystem for progress, innovation and for the benefit of everyone with a rare disease. We support rare diseases stakeholders by funding research, bringing together data resources & tools, providing dedicated training courses, and translating high quality research into effective treatments.
Research line: Reusable health data
Staff: Nirupama Benis, Ronald Cornet, Shuxin Zhang
The EQUAL study, part of the ERA-EDTA registry, is an European prospective cohort study in elderly patients in chronic kidney disease stage 4. EQUAL aims to study how the level of renal function, and uraemic signs and symptoms can be used to determine when dialysis should be initiated in elderly patients.
Research line: Epidemiology of kidney disease
Staff: Nick Chesnaye, Megan Astley, Kitty Jager
Website: EQUAL study website.
Model explainability and transparency are requirements in healthcare applications. This is especially important when proposing models for healthcare that consume or generate natural language inputs such as free-text clinical notes. The major trend in explainability research in artificial intelligence resorts to the post-hoc explainability of black-box models, which are methods that try to approximate how a black-box model produces their predictions. However, we would like to build models that are inherently explainable and transparent. In this project, we propose not only post-hoc explainability methods for NLP, but also NLP methods that are explainable by design.
Research line: Methods in Medical Informatics
Staff: Nishant Mishra, Iacer Coimbra Alves Cavalcanti Calixto, Ameen Abu-Hanna
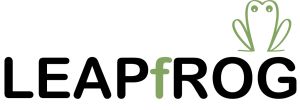
People with multimorbidity suffer from suboptimal pharmacotherapy due to insufficient knowledge to guide prescribing decisions. Leveraging routinely collected data in Electronic Health Records (EHRs) using machine learning (ML), have been widely advocated to close this knowledge gap and provide missing insights on safety and effectiveness of drugs. However, EHR data suffer from many quality issues. In addition, there are still significant limitations in the introduction of ML models to support in prescribing decisions, due to the lack of transparency in how model outputs are generated (the "black box” dilemma), and lack of logical reasoning (models not informed by causal domain knowledge).
Therefore, LEAPfROG aims for scientific breakthrough in ML application in healthcare by addressing the aforementioned limitation and creating a medication safety learning system. A system powered by novel ML-powered tools capable to provide trustworthy and explainable causal suggestions. The value of the ssystem will be demonstrated via clinically relevant and urgent use case of drug-induced kidney diseases (DIKD) in patients with chronic kidney disease (CKD). Patients with CKD are amongst the most complex and multimorbid. By providing missing insights on DIKD in patients with CKD via PIL, LEAPfROG will contribute to safer and more effective pharmacotherapy in these patients. Aiming to increase quality of life of patients with CKD and decrease healthcare costs related to medication harm
Timespan: 2022 - 2027
Research line: PharmacoInformatics
Website: LEAPfROG
This project studies the various ways to transform the NICE ICU quality registration data structure in order to lower the registration burden on intensivists, to increase data collection from monthly to daily, to make the NICE data reusable for researchers, and to facilitate federated analysis without having to share data directly. Various standardization solutions are explored, such as the data structure standard OMOP CDM and the data exchange standard HL7 FHIR. These are then evaluated in collaboration with international organizations such as European Health Data & Evidence Network (EHDEN), and the Critical Care Asia Africa (CCAA) registry network. By making following the Findable, Accessible, Interoperable and Reusable (FAIR) principles we hope to make the NICE data easier to share, use, and reuse.
Research lines: Quality of Care (IT Systems) & Reusable Health Care
Staff: Daniel Püttmann, Nicolette de Keizer, Ferishta Raiez, Ronald Cornet
In the ICU, 71% of the patients receive antibiotics. Of these antibiotics, 30% to 60% is prescribed unnecessary, inappropriate, or suboptimal.
Audit & Feedback is proven to be effective in stimulating quality improvement, therefore the NICE2Improve dashboard was developed. This dashboard includes actionable quality indicators and a toolbox with suggestions of improvement actions to support ICU staff on how they can increase appropriate antibiotics use. The purpose of this research is to pinpoint areas where specific ICUs might experience barriers to quality improvement, to devise a method for creating a tailored toolbox, and to evaluate the effectiveness of the tailored toolbox in enhancing antibiotic use in the ICU.
Research line: Quality of care (IT systems)
Machine learning (ML) models have made remarkable advancements in analyzing medical data, yielding impressive results. However, a significant limitation lies in their performance, which is primarily optimized for data from the training distribution and can degrade when the model is used on a different distribution. As we aim to deploy these ML models in real-world healthcare scenarios, ensuring their reliability becomes of utmost importance. Consequently, it is crucial to devise a method that can effectively detect samples that lie outside the training distribution before making potentially erroneous predictions on them. By doing so, we can proactively avoid erroneous predictions on out-of-distribution (OOD) data.
In this project, we address this specific challenge within the context of medical tabular data. Our primary objective is to investigate and develop a robust method for identifying OOD data points in real time. By developing effective solutions to detect OOD data in medical tabular datasets, we can elevate the reliability and applicability of ML models in real-world healthcare settings. This could potentially lead to improved patient outcomes and enhanced decision-making processes, as healthcare professionals can trust the model's predictions and be alerted when encountering unfamiliar or OOD cases. Ultimately, our research aims to bridge the gap between ML advancements and practical healthcare implementation, fostering a more reliable and secure healthcare AI ecosystem.
Research line: Methods in Medical Informatics
Staff: Giovanni Cinà, Mohammad Azizmalayeri, Ameen Abu-Hanna
This project is a consortium with the medical device company Pacmed and it aims at supporting the decisions of intensive care clinicians dealing with treatments of variable length. Such treatments are difficult to administer because it is hard to understand when is the optimal time to stop treatment; for instance with mechanical ventilation, it can be hard to judge when the patient can be safely extubated. The work package entrusted to KIK concerns the study of causal inference techniques to assess the effect of such dynamic treatments. These solutions will then be tested on real-world data leveraging the ongoing collaboration between Pacmed and the Santeon hospitals, enabling a quick iteration and a speedy trajectory towards the improvement of current hospital processes.
Timespan: 2022-2026
Research line: Methods in Medical Informatics
Staff: Giovanni Cinà, Sagar Simha, Ameen Abu-Hanna
PaLaDIN aims to accelerate the development of effective treatments and to establish best-practice diagnosis and care for neuromuscular disease patients worldwide by harnessing patient input and patient-generated health data to improve decision making, outcomes and healthcare solutions. To this end, PaLaDIn will develop and operate a collaborative, inclusive system that collects patient-reported outcome and experience measures, and aligns these with clinical data to advance treatments, diagnosis, and care by providing holistic, patient-level data.
Research line: Reusable health data
Staff: Nirupama Benis, Ronald Cornet, Martijn Kersloot
Adverse drug events (ADEs) are a major public health concern. In the Netherlands, ADEs are a leading cause of preventable patient harm in hospitalized patients, and their reduction is needed but so far unsatisfactory. Currently, information about ADE occurrence mainly comes from voluntary reporting. However, research shows that fewer than 1% of ADEs are reported in this way. ADEs are often documented by physicians in clinical notes as part of routine care, and clinical pharmacists would need to manually analyze vast amounts of clinical notes to gain a thorough insights on ADE occurrence. This is prohibitively time consuming and not future-proof. An automated method for ADE detection from clinical notes would support clinical pharmacists in their crucial role as medication safety gatekeepers. Natural language processing (NLP), a computerized approach to analyze text data, has shown promising results for the purpose of ADE detection from clinical notes. However, most NLP methods available were develop on clinical text in English.
Within the hospitalized patients, patients admitted to the Intensive Care Unit, are most prone to ADEs due use of multiple, high-risk drugs. At present, a routine identification of Adverse Drug Events (ADEs) is not available for Dutch Intensive Care Units (ICU). This hampers timely ADE detection. Especially drug-induced acute kidney injury (DAKI) presents serious concerns for ICU patients. Therefore, the main goal of the REMEDY study is to develop and validate an automated method to detect ADEs in Dutch clinical notes using Natural Language Processing (NLP).
Timespan: 2020-2024
Research line: PharmacoInformatics
Website: REMEDY
The current best practice approach for the diagnosis of adverse drug events (ADEs) in hospitalized patients relies on a manual procedure, including a full patient chart review and a formal causality assessment by multiple medical experts. The resource intensive character of this approach, hampering large scale medication safety monitoring and use in daily, often busy, clinical practice. Furthermore, this approach also suffers from low inter-rater reliability and a high risk of bias. Novel methods for the diagnosis of ADEs are therefore urgently needed, and artificial intelligence may aid through automated, data-driven approaches. Within the hospitalized patients, patients admitted to the Intensive Care Unit, are most prone to ADEs due use of multiple, high-risk drugs. Especially drug-induced acute kidney injury (DAKI) presents serious concerns for ICU patients. Therefore, the aim of the RESCUE project is to develop and validate an automated DAKI causal algorithms for ICU patients by utilizing advanced machine learning techniques and best-practices for causal inference using observational routinely collected ICU data. If proven successful, the developed automated and data-driven approach may be applicable for any other ADEs.
Timespan: 2020-2024
Research line: PharmacoInformatics
Website: RESCUE
For health outcomes, socioeconomic status (SES) is an important determinant. Generally, a lower SES is associated with more adverse healthcare outcomes. It is unclear whether this phenomenon is present in patients admitted to the intensive care unit (ICU), especially in The Netherlands.
Therefore, this nationwide observational cohort study will investigate SES in the total Dutch ICU population and across regions, the association between SES and (hospital/long-term) mortality, and its effect on individual estimated mortality risks. Depending on the outcomes, we will try to improve the case-mix model (APACHE IV) for estimating individual mortality risks and examine its influence on benchmarking Dutch ICUs. We will use a composited (occupation, income, education) SES score per household and per postal code to identify possible differences between these scores.
Research line: Quality of care (IT systems)