Methods in Medical Informatics
Study and apply data methods. We study and explore new AI and other methods of analyzing data, which may also include free text, within the healthcare sector. We generate predictions with these data and identify causes of diseases or other outcomes. Within this research line, we also develop computer programs that can assist healthcare providers in making decisions. Our main clinical partners are from the departments of general practice, geriatrics, perinatology, cardiology, and intensive care.
Scientific projects
This project is a consortium with the medical device company Pacmed and it aims at supporting the decisions of intensive care clinicians dealing with treatments of variable length. Such treatments are difficult to administer because it is hard to understand when is the optimal time to stop treatment; for instance with mechanical ventilation, it can be hard to judge when the patient can be safely extubated. The work package entrusted to KIK concerns the study of causal inference techniques to assess the effect of such dynamic treatments. These solutions will then be tested on real-world data leveraging the ongoing collaboration between Pacmed and the Santeon hospitals, enabling a quick iteration and a speedy trajectory towards the improvement of current hospital processes.
Timespan: 2022-2026
Research line: Methods in Medical Informatics
Staff: Giovanni Cinà, Sagar Simha, Ameen Abu-Hanna
Machine learning (ML) models have made remarkable advancements in analyzing medical data, yielding impressive results. However, a significant limitation lies in their performance, which is primarily optimized for data from the training distribution and can degrade when the model is used on a different distribution. As we aim to deploy these ML models in real-world healthcare scenarios, ensuring their reliability becomes of utmost importance. Consequently, it is crucial to devise a method that can effectively detect samples that lie outside the training distribution before making potentially erroneous predictions on them. By doing so, we can proactively avoid erroneous predictions on out-of-distribution (OOD) data.
In this project, we address this specific challenge within the context of medical tabular data. Our primary objective is to investigate and develop a robust method for identifying OOD data points in real time. By developing effective solutions to detect OOD data in medical tabular datasets, we can elevate the reliability and applicability of ML models in real-world healthcare settings. This could potentially lead to improved patient outcomes and enhanced decision-making processes, as healthcare professionals can trust the model's predictions and be alerted when encountering unfamiliar or OOD cases. Ultimately, our research aims to bridge the gap between ML advancements and practical healthcare implementation, fostering a more reliable and secure healthcare AI ecosystem.
Research line: Methods in Medical Informatics
Staff: Giovanni Cinà, Mohammad Azizmalayeri, Ameen Abu-Hanna
MediSpeech will present an integrated, digital IT ecosystem for automated medical reporting with proven added value in the clinical practice, in the shape of platform infrastructure that supports the transformation of the organisation-centred healthcare model into a patient/doctor-centred model.
The proposed solution will go along with healthcare’s mission of bettering patient care through the use of assistive, digital tools. This will be achieved through the integration of the innovative technologies proposed with the reference to the current SotA:
(1) AI-powered speech recognition for less-error rate and accurate dealing with the idiosyncrasies of an individual’s speech patterns,
(2) Data interoperability and harmonisation allowing combining multiple services with complementary functionalities,
(3) Technology-advanced clinical decision support system based on hybrid recommendations,
(4) Medical reporting providing past data-driven knowledge needed to boost effectiveness and reduce medical complication rates while attaining performance targets.
Research line: Methods in Medical Informatics and Natural Language Processing
Staff: Iacer Coimbra Alves Cavalcanti Calixto, Ameen Abu-Hanna, Henk Marquering, Azam Nurmohamed
Model explainability and transparency are requirements in healthcare applications. This is especially important when proposing models for healthcare that consume or generate natural language inputs such as free-text clinical notes. The major trend in explainability research in artificial intelligence resorts to the post-hoc explainability of black-box models, which are methods that try to approximate how a black-box model produces their predictions. However, we would like to build models that are inherently explainable and transparent. In this project, we propose not only post-hoc explainability methods for NLP, but also NLP methods that are explainable by design.
Research line: Methods in Medical Informatics
Staff: Nishant Mishra, Iacer Coimbra Alves Cavalcanti Calixto, Ameen Abu-Hanna
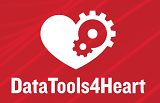
Within the datatools4heart project, we work on the co-creation, development and demonstration of a comprehensive, federated, privacy-preserved cardiology data toolbox. This toolbox includes standardized data ingestion and harmonization tools, multilingual natural language processing and federated machine learning and data synthesis methods. Additionally, with the development of a public data catalog combined with virtual assistants, scientist and clinicians can navigate easily through large-scale multi-source cardiology electronic health record data, while complying to the European regulations and data standards.
Amsterdam UMC participates as one of the clinical sites, focusing on data harmonization, adoption of a federated learning platform and free-text analysis using natural language tools.
Research line: Methods in Medical Informatics
Staff: Noman Dormosh, Iacer Coimbra Alves Cavalcanti Calixto, Ameen Abu-Hanna, Machteld Boonstra
Website: www.datatools4heart.eu
Patients admitted to the Intensive Care Unit (ICU) are often treated with multiple high-risk medications. Yet, our knowledge of safety and effectiveness of pharmacotherapy in ICU patients is limited. Consequently, over- and underprescribing of indicated medications, and inappropriate choice of medications, frequently occur in ICU patients.
Medication Recommender Systems (MRS) are novel systems that can assist physicians with the selection of appropriate medications and with flagging inappropriate medications by uncovering patterns and exploiting similarity among patients’ and medications’ data. MRS can provide personalized suggestions, which are inherently more useful, relevant and efficient.
The main objectives of this project are:
1) building a proof-of-concept MRS for Amsterdam UMC ICU patients, and
2) investigating its potential value compared to the current rule-based CDSS.
We will leverage both rule-based methods using the problem list, and machine learning, deep learning, and natural language processing to provide personalized suggestions by uncovering patterns in the medication data, problem-list data, laboratory findings, and notes.
Research line: Methods in Medical Informatics
Staff: Chao Zhao, Iacopo Vagliano, Ameen Abu Hanna, Joanna Klopotowska
Unstructured data is guesstimated to account for 80% of all patient data. Nonetheless, unstructured data is currently severely underused in healthcare because it is noisy, hard to interpret, and privacy-sensitive. In this project, we develop human-centric responsible Natural Language Processing (NLP) and Machine Learning (ML) methods that will allow clinicians and patients to safely tap this unstructured data’s potential.
Our proposed methods have a focus on the Dutch healthcare ecosystem and are tailored to support research, education, and patient care by promoting explainable prediction models, ensuring fairness and patient privacy, preventing bias, and coping with data scarcity.
The CaRe-NLP project kicks-off in April 2024 and will run for 5 years until April 2029.
Research line: Methods in Medical Informatics en Natural Language Processing
Staff: Nishant Mishra, Heloisa Oss Boll, Xinlan Yan, Iacer Coimbra Alves Cavalcanti Calixto, Ameen Abu-Hanna
Website:https://nlp4health-lab.github.io/projects/carenlp_project/
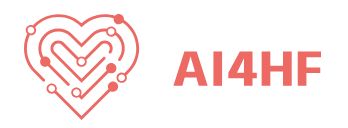
The AI4Hf project focusses on co-design, development, evaluation and exploitation of integrative and thrustworthy artificial intelligence solutions for personalized heart failure risk-assessment. These tools will be trained using large-scale complementary multi-source cardiovascular data including cardiac imaging, -biomarkers, electrocardiography and free text data. Within the project, great emphasis is placed on the concept of trustworthy and inter-dicilplinary tool design, to ensure that developed tools are trusted, approved and deployed in a real world setting and providing clear benefit for patients, cardiologists, physicians and health-care providers.
The consortium is led by Amsterdam UMC and Amsterdam UMC focusses on defining requirements for the development of trustworthy AI, model development and explainability.
Research line: Methods in Medical Informatics
Staff: Giovanni Cinà, Machteld Boonstra
Website: www.ai4hf.com
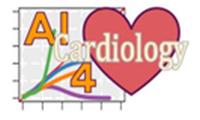
AI4Cardiology is a collaborative data science effort performed by a multidisciplinary team to explore the potential of machine learning in the field of cardiology. By delving deep into complex, real-world cardiac datasets, we aim to uncover valuable insights and positively impact clinical decision-making.
Time span: 2021-2024
Research line: Methods in Medical Informatics
Staff: Tseko Yordanov, Anita Ravelli, Ameen Abu-Hanna
Staff involved